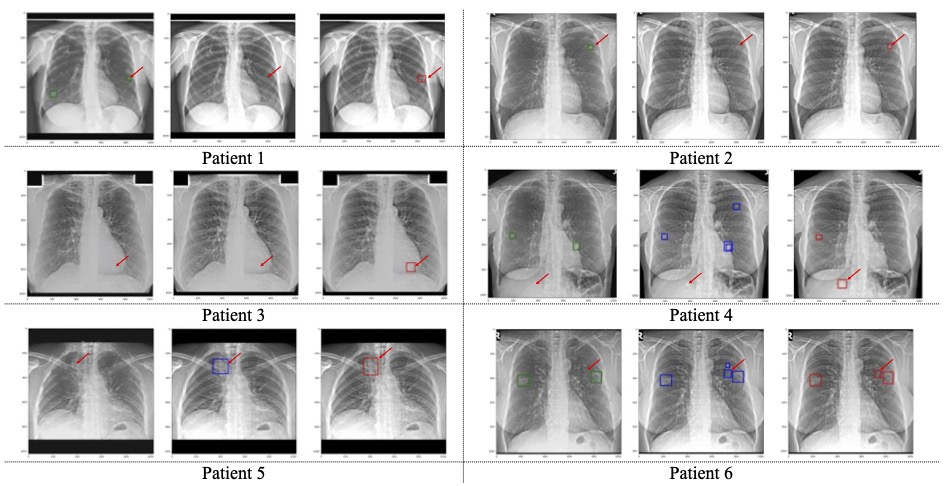 |
Deep Learning Based Object Detection of Lung Nodule with Style
Transfer Network Assisted Bone Suppression Techniques for Chest X-Ray (CXR) Analysis.
Di Xu, Qifan Xu, Dan Ruan, Fabien Scalzo and Ke Sheng
This is a better solution compared to the NODE21 "Tran-QWin-Bag". Submitted to MICCAI 2022.
|
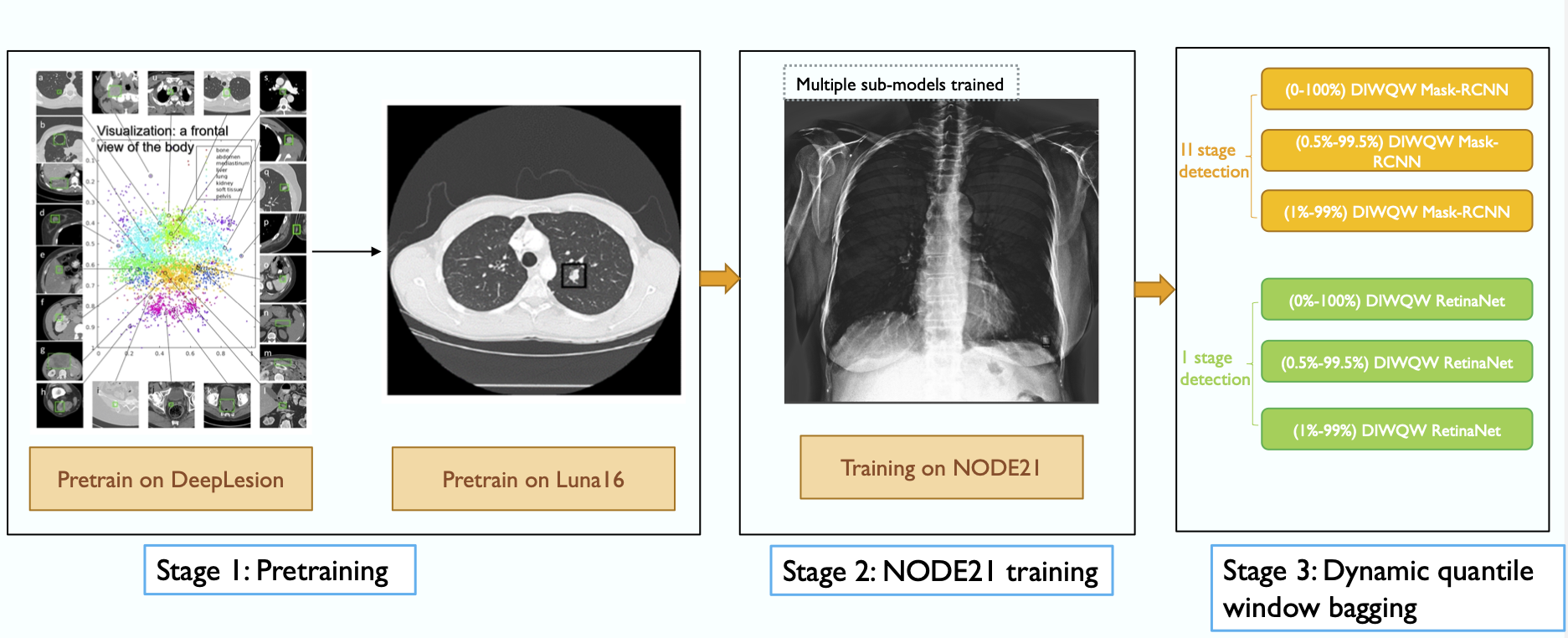 |
Trans-QWin-Bag: A Transfer Learning and Quantile Window Bagging
Assisted Object Detection Framework for Chest X-ray (CXR) Nodule Detection.
Di Xu, Fabien Scalzo and Ke Sheng
This is the solution ranking #3 on the NODE21 MICCAI challenge
|
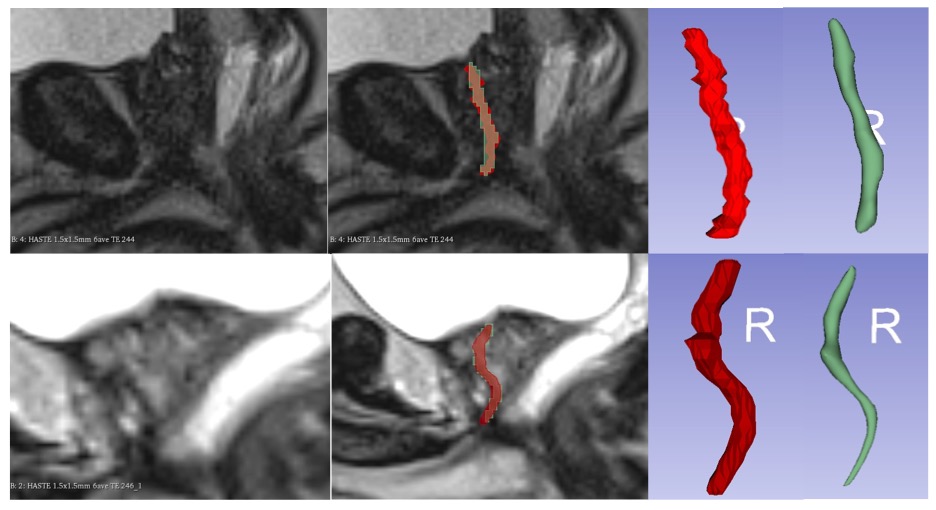 |
3D nnU-Net Inspired Auto Segmentation Framework of Prostatic Urethra Using MR-Guided
Radiation Therapy (MRgRT) On-Board MRI: an Efficient Approach to Assist Quantification of Dose
Distribution in Prostate Cancer Radiotherapy.
Di Xu, Ting Martin Ma, Ricky Savjani, Minsong Cao, Yingli Yang, Amar U. Kishan, Fabien Scalzo and Ke Sheng
Submitted to Red Journal
|
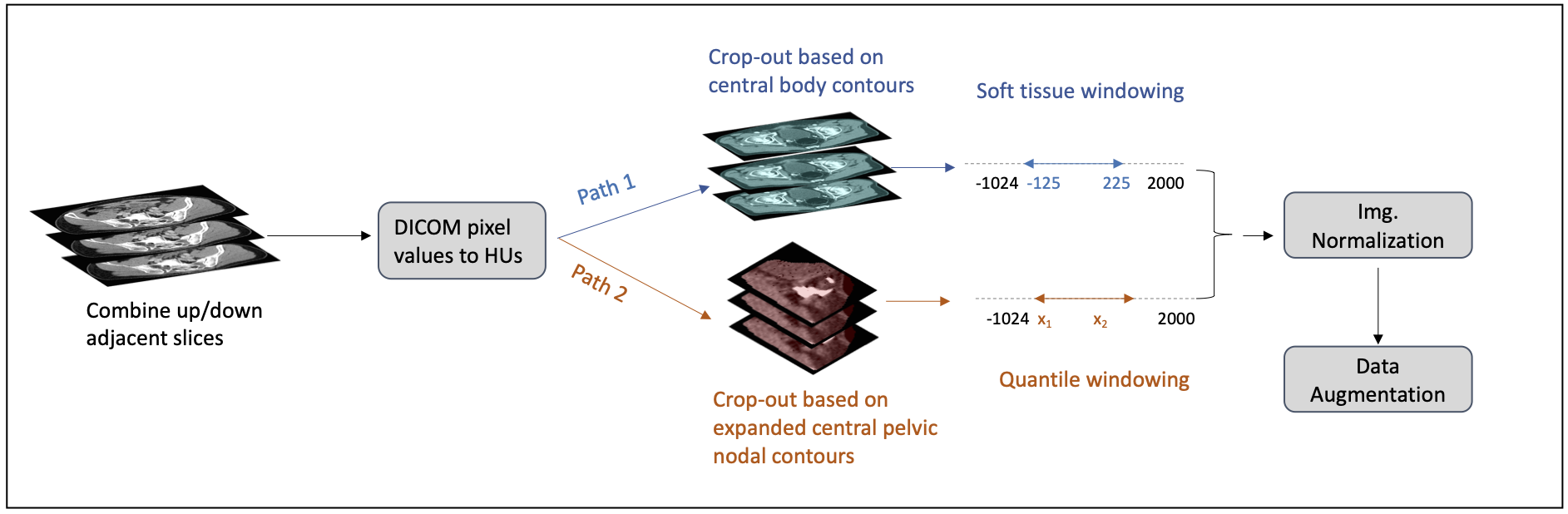 |
Mask R-CNN Assisted 2.5D Object Detection Pipeline of
68Ga-PSMA-11 PET/CT-positive Metastatic Pelvic Lymph Node After Radical Prostatectomy.
Di Xu, Ting Martin Ma, Minsong Cao, Amar U. Kishan, Nicholas G. Nickols, Fabien Scalzo and Ke Sheng
Submitted to Artificial Intelligence in Medicine
|
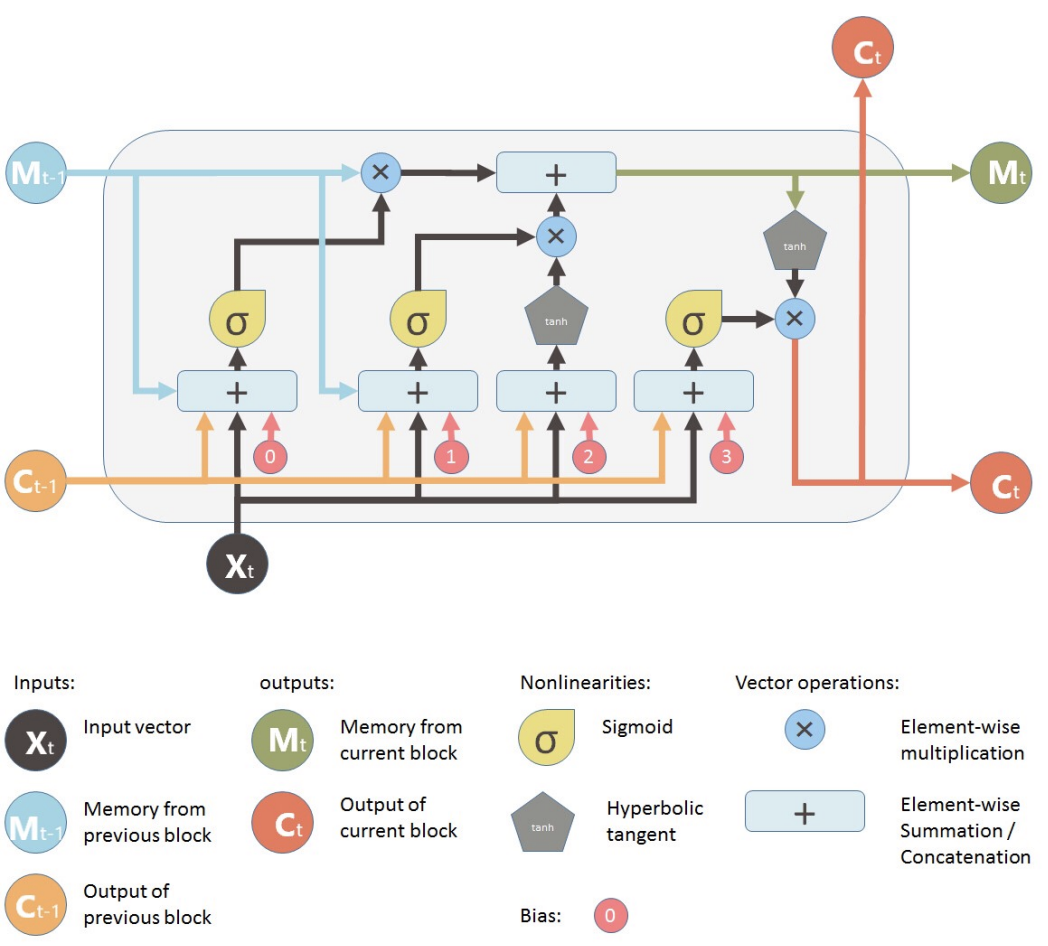 |
LSTM-Assisted Evolutionary Self-Expressive
Subspace Clustering
Di Xu, Tianhang Long and Junbin Gao
International Journal of Machine Learning and Cybernetics 2021
pdf |
abstract
Massive volumes of high-dimensional data
that
evolves over time is continuously collected by contemporary
information processing systems, which brings up the problem
of organizing this data into clusters, i.e. achieve the purpose
of dimensional deduction, and meanwhile learning its temporal
evolution patterns. In this paper, a framework for evolutionary
subspace clustering, referred to as LSTM-ESCM, is introduced,
which aims at clustering a set of evolving high-dimensional data
points that lie in a union of low-dimensional evolving subspaces.
In order to obtain the parsimonious data representation at each
time step, we propose to exploit the so-called self-expressive
trait of the data at each time point. At the same time, LSTM
networks are implemented to extract the inherited temporal
patterns behind data in an overall time frame. An efficient
algorithm has been proposed based on MATLAB. Next, experiments are carried out on real-world
datasets to demonstrate the
effectiveness of our proposed approach. And the results show that
the suggested algorithm dramatically outperforms other known
similar approaches in terms of both run time and accuracy.
|
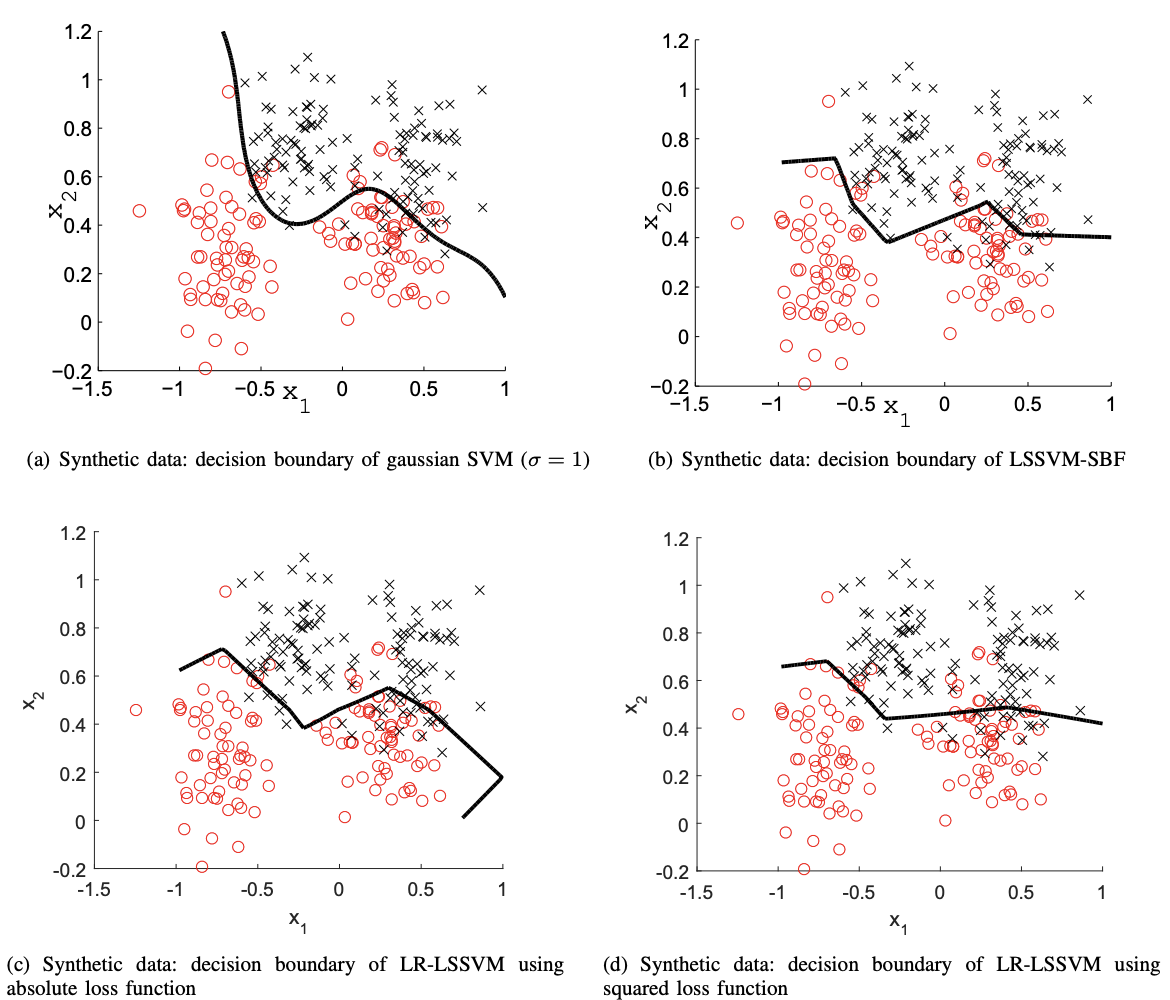 |
Sparse Least Squares Low Rank Kernel Machines
Di Xu & Manjing Fang, Xia Hong and Junbin Gao
ICNIP 2019
pdf |
abstract
A general framework of least squares
support vector
machine with low rank kernels, referred to as LR-LSSVM, is
introduced in this paper. The special structure of low rank
kernels with a controlled model size brings sparsity as well
as computational efficiency to the proposed model. Meanwhile,
a two-step optimization algorithm with three different criteria
is proposed and various experiments are carried out using
the example of the so-call robust RBF kernel to validate the
model. The experiment results show that the performance of the
proposed algorithm is comparable or superior to several existing
kernel machines.
|
|